Improved Forecasting for Auto Service Retailers
Financial due diligence requires the right balance between looking through the rearview mirror and the windshield.
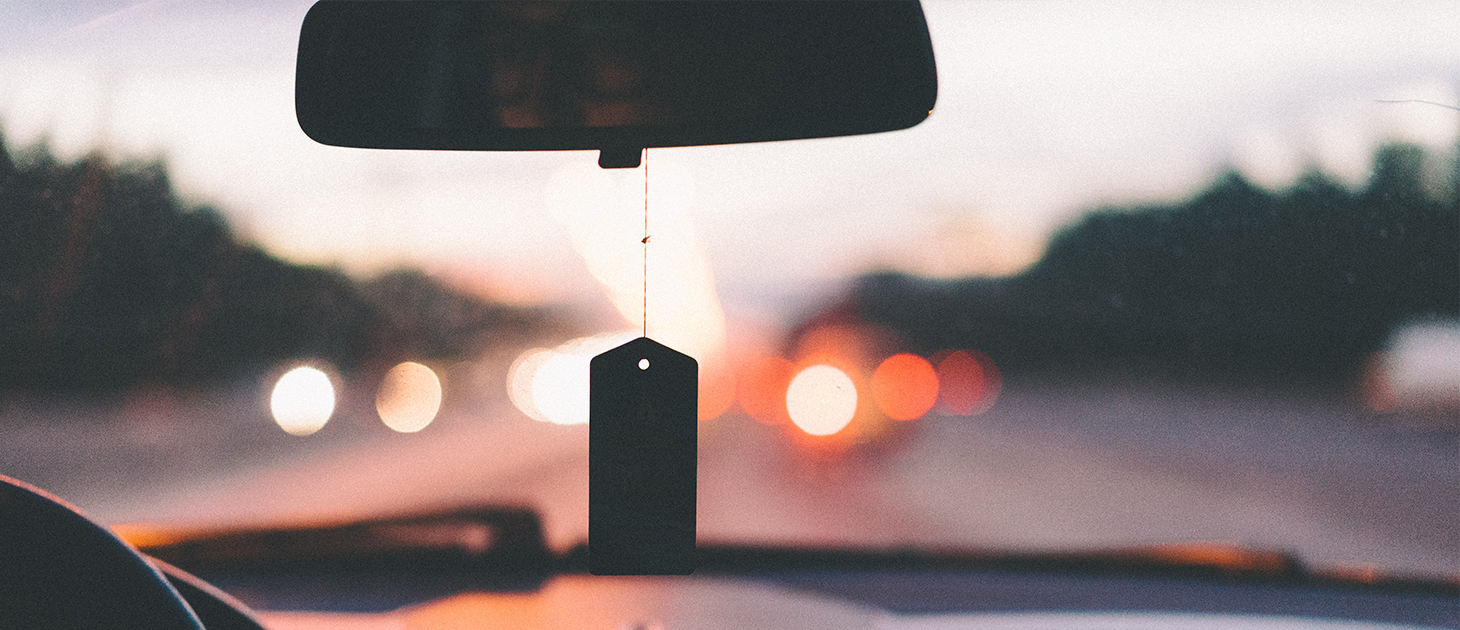
This article is sponsored by Dixon Hughes Goodman LLP.
This story originally appeared in the May/June 2020 print edition of Middle Market Growth magazine. Read the full issue in the archive.
Forecasting revenue and EBITDA growth linked to acquisitions or greenfield expansion is both an art and a science, and it can quickly become one of the most scrutinized areas during financial due diligence. In order to make pro forma earnings adjustments meaningful and identify additional sources of value, there needs to be a sophisticated approach that’s deeply grounded in thoughtful data analytics.
Financial due diligence requires the right balance between looking through the rearview mirror and the windshield. Pro forma adjustments are particularly relevant for multi-unit retailers in the auto service industry that have either experienced growth via geographic expansion, or that are considering expansion based on the investment thesis. Robust data analytics can help accurately depict the “certainty of the future” and better inform the due diligence process and findings for both buyers and sellers of multi-unit retail businesses.
Pro forma adjustments have historically been calculated based on past performance of the acquired locations or on general assumptions that greenfield stores may have operations comparable to their mature-store brethren. However, both methods rely on a limited set of historical data points.
Microsoft Excel has been used as the primary tool for analyzing the data. While a good analytical framework can be built in Excel, there are other advanced products available. Alteryx, TIBCO Spotfire and R are among the tools with enhanced data extraction, transformation and analysis components that significantly reduce the time needed to analyze large and varied amounts of data and to enhance the pro forma adjustment process.
Combining internal company information with external data can help mitigate risk and identify value levers. Examples of internal company data include location and site-specific attributes, historical financial performance and key performance indicators such as volume, price, product and margin trends. External data consists of demographic, geographic and competitor locations that can be found in census data or other sources in the public domain, or through third-party data providers. A modeling approach such as regression analysis can then be used to closely predict the revenue-to-EBITDA ratio for various locations upon maturity.
Every modeling approach has limitations. In the case of regression analysis, inadequate data or limited operational insight may be insufficient to support a robust analysis on existing or mature sites, or provide misleading conclusions. Exercise caution and consult with subject matter experts to understand and model the data.
Investing in data analytics efforts can better position sellers to maximize the transaction value by identifying defensible adjustments that can stand up to enhanced scrutiny during due diligence. Meanwhile, buyers can benefit from being more prepared to proceed, renegotiate, restructure or withdraw from a potential transaction or high-multiple purchase price.
There is additional value for acquirers that are armed with data analytics after the deal closes and during the integration and value-creation phases. This approach can be used to identify underperforming locations to inform turnaround procedures or to identify markets for future expansion. In today’s competitive marketplace, the buyers and sellers who successfully drive a deal forward are those who understand the value of advanced analytics and data.
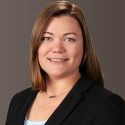
Kate Leverone is managing director, private equity and transaction advisory services, for Dixon Hughes Goodman LLP. She is based in the firm’s Atlanta office.